When ChatGPT first hit the market its human-like responses were astonishing, yet somewhat eerie. Many thought, “It communicates just like me.” It follows that the next logical thought was, “It’s going to take over my job.” However, the fear that AI-driven tools will replace human software testers is unfounded.
AI will certainly impact testing jobs, including market expectations, skillsets, and required knowledge. Only individuals who invest in acquiring new competencies — not just new programming languages, but also an understanding of the latest advancements in AI and machine learning — will stay ahead in a highly competitive market. The number of testing jobs will probably increase, and so might your paycheck.
In short, highly experienced testers who embed AI in their daily work and possess expertise in testing GenAI systems will be highly sought after — so don’t panic. Read on to learn how AI is evolving the industry, and what you can do today to stay competitive.
In a nutshell: The impact of AI on software testing jobs
AI won’t reduce the number of testing jobs. On the contrary, there will be an increased demand for testers with specific skills, so the bar will be raised. What specific skills are we talking about?
In general, the future belongs to AI-assisted testers — professionals who apply AI to enhance overall productivity and efficiency. These individuals are likely to enjoy greater job stability, career progression, and rewards.
Generally, there will be less demand for people who can complete entry-level work such as creating extensive tests based on requirements. The market will pivot towards viewing experienced testers as capable of complex, higher-order thinking. This shift is critical because despite its advancements, AI remains prone to errors. Thus, expertise in testing and ensuring the reliability of GenAI systems will become increasingly important.
Finally, we have a third wave of change. This involves organizations who toy with the idea of having AI autonomously develop and test applications from the ground up, potentially bypassing human intervention. However, the effectiveness, cost-efficiency, and quality outcomes of such endeavors are still under scrutiny. Will these approaches deliver tangible benefits, or will they necessitate human intervention to rectify the shortcomings? The verdict on this is still pending.
For better or worse, AI is reshaping the QA industry. The choice is yours: ignore the inevitable or embrace the change to secure your place in the future of testing. Your decision will significantly influence your career trajectory, job security, and compensation.
Reactions to AI
When ChatGPT first appeared, it was downloaded by 100 million people within just two months. This made it the fastest-growing consumer technology in history — a milestone that took Facebook four years to achieve. Despite the widespread and virtually effortless access to ChatGPT and numerous other AI-based applications, the overall adoption rate of AI remains surprisingly modest. This phenomenon can be partly explained by Geoffrey Moore’s renowned technology adoption curve outlined in “Crossing the Chasm,” which suggests that the mainstream market typically adopts new technologies at a slower pace. Rest assured that AI has managed to “cross the chasm,” but does this equate to universal adoption among technology enthusiasts?
We highlight this to showcase the emergence of two distinct groups in response to AI:
- those who resist AI, criticizing it for not meeting expectations or denying its potential impact, and
- those with an open-minded, optimistic, or early-adopter mentality who embrace, experiment with, and adapt to AI.
In her book “Mindset: The New Psychology of Success,” Carol Dweck labels these groups as ‘fixed versus growth’ mindsets. Essentially, your stance towards AI could dictate your ability to stay ahead of the game. Individuals who neglect to integrate AI into their daily work are at risk of missing out on substantial productivity boosts and failing to acquire vital new skills, simply because they aren’t using the darn thing. If you prefer to avoid the new wave of technology, that’s your prerogative—there’s no obligation to adopt every innovation. However, for those eager to excel and secure a competitive edge in a rapidly evolving market, the time to embrace and understand AI is now, not later.
Before we explain how it will impact the market and what this means for you, let’s unpack first how AI is already being adopted by testers today, and what early trends show.
How GenAI can assist testers today
Today’s GenAI will change everything because it has three new magic properties:
- It knows almost everything. It possesses an unparalleled breadth of knowledge, having digested the entirety of the internet, including the most comprehensive testing manuals, as well as a myriad of blog posts, tutorials, and educational videos covering quality assurance and software testing. This extensive learning allows it to grasp and contribute to discussions across the full spectrum of testing topics.
- It is generative. GenAI has the remarkable ability to produce new content in text form, including test cases, test plans, strategies, risk analyses, and defect reports. Unlike old-school Google search, it doesn’t find things already written by humans — it generates new things.
- It is multimodal. It’s not just capable of generating new content in text format, but also in image, audio, and video format. This opens Pandora’s box of new avenues for application scanning, action execution, and automation steering, presenting a wealth of unexplored opportunities for testing practices.
For this reason, testers are already using AI, chatbots, or new AI-assisted testing tools in various ways to speed up their work. From autogenerating test cases to summarizing requirements, self-healing, generating test data, detecting defects, or smart impact analysis, the applications are vast. Forrester predicts 15% productivity gains for testers. Others, like developers using GitHub’s Copilot AI assistant, are 55% more productive.
The point is that to survive this transition, you should stay up to date on the latest use cases. Doing so not only ensures job security, but significant rewards and compensation. For more use cases, read our blog Myth vs. Reality: 10 AI Use Cases in Test Automation today.
At the heart of AI: Testing
Once you start using AI tools, you’ll soon discover some common traits: they make mistakes, they are arrogant, and they don’t question things much. In a sense they are too human, paradoxically speaking. The reality of the market today is that AI is very much prone to error. AI really needs human guidance. Not only does it need human guidance, but it also needs testing.
At the heart of AI is the testing discipline. Think about all the times you say, “let’s test ChatGPT to see if this works.” “Let’s test this new AI-powered phone to see if it can book my next holiday.” “Let’s test to see if we can trick ChatGPT to expose personal information.” By and large, AI is about testing. If anything, AI will elevate the testing discipline because it’s so core and fundamental to AI. Human testers are at the center of this shift. We need human testers more than ever to steer that process.
Testing AI-based systems: A whole new world
You may have heard of the phrase “red teaming.” The Biden administration recently released an executive order stating that certain high-risk generative AI models undergo red teaming, which is loosely defined as “a structured testing effort to find flaws and vulnerabilities in an AI system.”
While there is no clear consensus on what red teaming means in practice, tech companies have already begun embracing the method for creating safe and trustworthy generative AI. Broadly speaking, red teams interact with generative AI systems to provoke them into generating malevolent prompts or producing harmful or inappropriate responses.
But what exactly comprises a red team? The Biden administration has directed efforts to systematically define not only the “who,” but also the “how” and “which” generative AI systems will be scrutinized, the types of risk assessments to be applied, and the procedures for documenting and gathering pertinent data.
Red teaming heralds a golden age for testing; it levels the playing field for testing GenAI systems. There are already numerous proactive, pioneering QA professionals who are at the forefront of these developments, identifying common and novel techniques for testing AI and helping to innovate the field.
The future tester: Implications for you and your job
The future tester, as previously outlined, is an AI-assisted tester. This professional harnesses AI as a smart assistant in their daily work, employing a “human → computer → human” workflow that significantly boosts the efficiency, throughput, and quality of testing activities. Humans and AI are more powerful working together than standing alone.
Furthermore, the future tester is someone who continuously explores news methods and techniques for testing AI systems. The focus is shifting from traditional application testing to the evaluation and monitoring of highly autonomous AI systems, which promise to significantly streamline the end-to-end testing process. While we haven’t fully realized this potential — given that AI, despite its early productivity benefits, still produces errors (for instance, GitHub’s Copilot yields code with security bugs and design flaws 40% of the time) — the trajectory is clear. The role of testers in closely monitoring and evaluating AI systems is becoming increasingly central and indispensable.
Expanding skillsets: Essential areas of focus
Below, we’ve outlined a list of essential competencies that will help you maintain a competitive edge.
- Data analysis: When you’re dealing with generative AI systems, remember that they generate a lot of data and sometimes it’s easy to get overwhelmed. Your job as a tester is to navigate this data deluge by identifying vulnerability and looking for ways to extract sensitive data like internal data, or PII data that may have inadvertently been incorporated into the LLM. A core component is to understand whether the AI system was trained on any internal or customer sensitive data, or whether APIs access any internal system. If so, testers must be adept in retrieving such information from AI systems.
- Testing techniques for AI systems: Staying updated with the latest methods to challenge AI systems is essential. For instance, “prompt overflow,” a technique where a red team intentionally overloads the system with a large input to disrupt its primary function, is a common tactic. Such actions can potentially repurpose the AI for unintended outcomes, from reciting Taylor Swift song lyrics (joke intended) to revealing sensitive information. Familiarity with industry-standard techniques for compromising AI capabilities is also necessary. Testing could involve assessing whether a bot designed for product recommendations could be manipulated to execute unauthorized actions, highlighting the importance of understanding these attack vectors.
- AI model monitoring: How do you ensure the overall quality of an AI system? One of the most important questions to ask before releasing any AI-infused application to the public is whether it does the job it was intended for. For instance, if a bot cannot answer basic questions, it cannot be deployed because it’s not doing its job and it can cause reputational damage. An essential step in this process involves establishing benchmarks for the AI system’s intended capabilities. These involve a set of questions or tasks your AI systems must successfully complete. Remember, you don’t start with building a bot and then verifying it against a benchmark. Benchmarking comes first, and bot development comes second. Work together with product or AI engineers to set those benchmarks and monitor the AI system regularly for errors, biases, or hallucinations in the outputs.
- Collaboration with data scientists and AI engineers: Work with AI engineering to understand the underlying models, how they are built, what data they were trained on, and what methods were used to get the AI system to complete a certain action. This can help you ensure accuracy, completeness, and testability of the AI systems.
- Spending more time with the customer or end-user: With the extra time at hand, it is important to understand the human component: the perceptual and emotional aspects of interacting with any application. At the core of this approach is adopting user experience testing. How do users implement the AI capability? What are their frustrations or expectations? Bringing this feedback to the product teams is instrumental to refining and improving the product.
Looking at quality holistically: Why AI alone cannot achieve it
Quality is an elusive term that can mean different things to different people. Quality can be affected by various external and internal factors, from the end-user’s perspective of the product to the way in which the business or team may work together to build and release a product, or even how the company culture, strategy, and leadership may impact the way software gets delivered (see image below). AI, despite its advances, cannot address the complex, multifaceted nature of quality on its own. For testers, the responsibility to ensure quality extends far beyond simple functionality checks of the software, and encompasses a holistic approach, especially when it involves testing AI systems. Therefore, testers should have specific skills and a holistic mindset to quality to be better equipped to release AI-based software. Necessary human characteristics include:
- Inquisitiveness and curiosity
- Lateral thinking skills
- Critical and analytical thinking skills
- Ability to zoom out (strategy) and zoom in (detail)
- Ability to grasp the domain and context
- Prior knowledge and experience
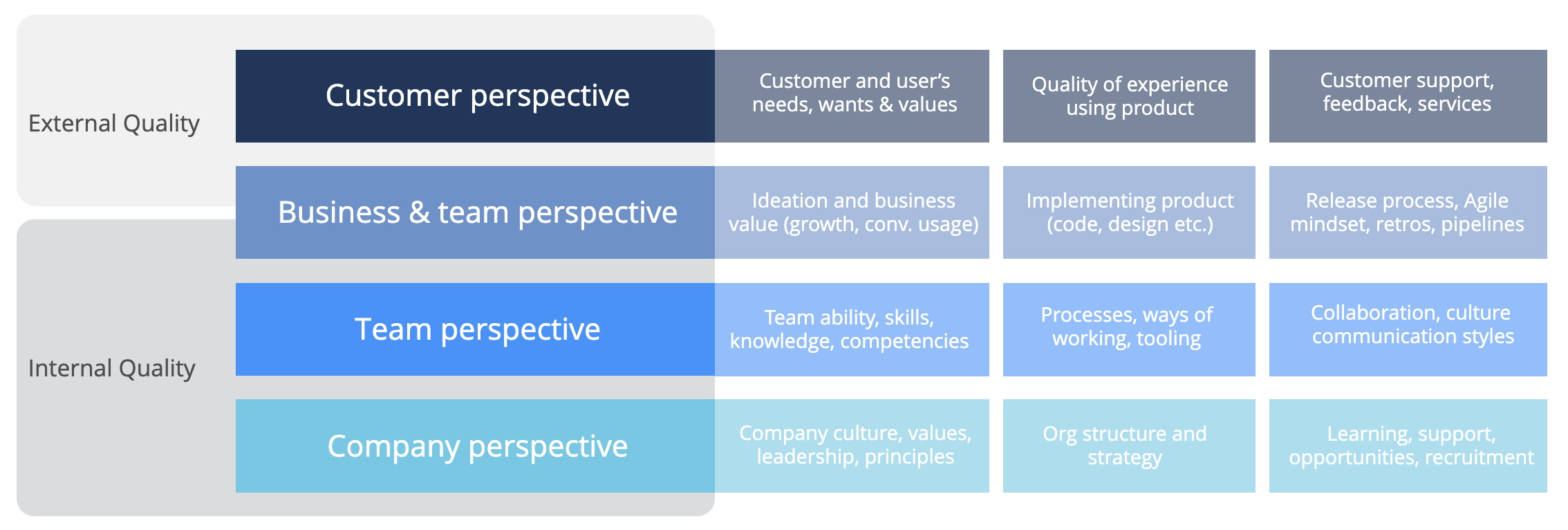
Concluding thoughts
By embracing lifelong learning, adopting new skills, and staying informed about the latest trends and techniques, testers can navigate the AI revolution in the software testing industry. Collaborating with cross-disciplinary teams and engaging with end users will further enhance the ability to ensure that AI systems are not only technologically advanced but also ethically sound, user-friendly, safe, and genuinely beneficial to society. In the era of AI, the human tester’s role is not diminished, but becomes more critical than ever. Testers are at the centerpiece of quality in this new AI frontier, ensuring that as we push the boundaries of what AI can achieve, we remain grounded in the principles of quality, responsibility, and human-centric design.
Want to learn more about our vision for the future of AI-driven testing? Check out our latest webinar.